Researchers at NASA’s Goddard Space Flight Center in Greenbelt, Maryland, are exploring the use of machine learning to speed up the analysis of Mars samples. The collection of data gathered by the rovers could be optimized to help scientists plan more efficient, time-efficient strategies for planetary missions.
Faster and more efficient Mars sample analysis
Dr. Xiang “Shawn” Li, a mass spectrometry scientist at Goddard Laboratory, mentioned that this algorithm can help to quickly filter the data and pinpoint those that may be more relevant or important for analysis. Initially, the algorithm will be tested with data from Mars, processed on a ground-based computer, using information collected by the Mars Organic Molecule Analyzer(MOMA) instrument.
MOMA is one of the main scientific instruments of the upcoming ExoMars mission, led by the European SpaceAgency (ESA). The Rosalind Franklin roverrover, scheduled for launch no earlier than 2028, aims to determine whether life ever existed on Mars. Once Rosalind Franklin collects and analyzes a sample with MOMA, the data will be sent to Earth, where scientists will use this data to decide on next steps.
According to Li, if “an analysis test” details signs of large, complex organic compounds mixed with specific minerals, decisions should be made: Conduct a more detailed study or assign the rover to collect a better sample, using its coring drill.
The importance of “machine learning
This branch of artificial intelligence allows computers to collect large amounts of data to identify patterns and make decisions. This process is useful in large and complex data sets for image and spectral analysis. Goddard data scientist and co-developer of the algorithm, Victoria Da Poian, explained that they have been successfully collecting laboratory data for more than a decade. The scientists train the algorithm with examples of substances that might be found on Mars and use it to generate predictions about the chemical composition of the samples.
In addition, Da Poian said that optimizing data analysis allows scientists to have more information and time to interpret the results, which facilitates quick reaction and efficient planning, as if they were present with the rover.
The Rosalind Franklin rover is special because it can drill up to 2 meters into the surface of Mars, while previous rovers only reached 7 centimeters. According to Li, organic materials on the surface of Mars are destroyed by radiation and cosmic rays, but at 2 meters deep, organic matter should be protected, which will allow MOMA to detect preserved ancient organic matter and advance the search for past life.
Future exploration of the solar system could be more autonomous. Li and Da Poian believe their algorithm could be useful in future missions to places such as Saturn’s moons Titan and Enceladus, and Jupiter’s moon Europa. Their long-term goal is to achieve more advanced scientific autonomy, where the mass spectrometer can analyze its own data and make operational decisions autonomously, increasing mission efficiency.
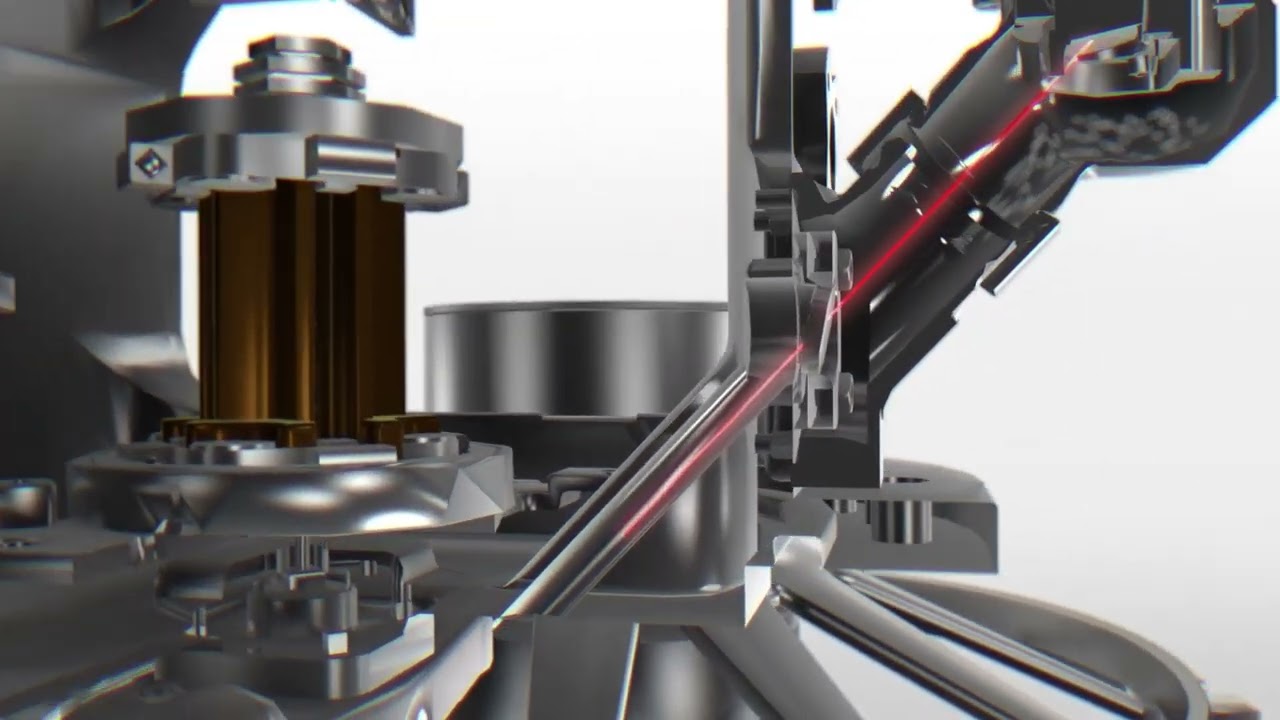
The Mars Organic Molecule Analyzer. Source: NASA
The MOMA project, led by the Max Planck Institute for Solar System Research(MPS) in Germany, has Dr. Fred Goesmann as principal investigator. NASA’s Goddard Institute developed and built the MOMA mass spectrometer subsystem, which will measure the molecular weights of chemical compounds in Martian samples.
Follow us on social networks and don’t miss any of our publications!
YouTube LinkedIn Facebook Instagram X
Source and photo: NASA