Introduction
Advanced Analytics is a set of techniques and tools that allow processing and analyzing large amounts of complex data in order to obtain valuable information for decision making. It is an approach that combines statistics, machine learning, and artificial intelligence to uncover hidden patterns and relationships in data, enabling companies to gain insights and insights that help them improve efficiency, make more informed decisions, and improve your overall performance.
Digital data and analytics can help achieve higher efficiencies through better planning, better combustion efficiency in power plants, and lower loss.
The application of this set of techniques refers to the application of advanced statistical and quantitative methods to extract information from the data. It involves the use of mathematical algorithms, machine learning, and other analytical techniques and methods including Big Data , Artificial Intelligence (AI), and Machine Learning , to analyze large volumes of and complex data to discover patterns, correlations, and trends that can help businesses to make more informed decisions.
Advanced analytics techniques include predictive modeling, machine learning, artificial intelligence, and natural language processing, among others. It also addresses how running analytics on IoT data can help control production, control potential data flooding, and drive business value for a company.
How advanced analytics is enabling real-time energy demand prediction and control?
Within advanced data analytics, three main types can be distinguished: descriptive analytics, diagnostic analytics, and predictive analytics.
Descriptive analysis involves creating a summary of historical data to provide useful information and prepare it for further analysis.
Diagnostic analysis is the phase in which data is processed to get to the root of a problem after finding a certain failure or anomaly. A report can be written that describes the failure and its possible solutions, or predictive or prescriptive analytics can be applied.
Predictive analytics provides tools to determine unknown or uncertain data about a business that requires a manual or costly process.
How is advanced analytics being used to integrate renewable energy into existing energy networks?
Smart grids are modernized energy systems that use digital communication technologies to manage the generation, distribution, and consumption of electricity intelligently and efficiently. These networks are designed to optimize energy use, reduce costs, and increase the reliability of power supply.
One of the main drivers of the need for digital transformation in smart grids is the increasing complexity of the power system. This intelligent connectivity is made up of a variety of distributed energy resources, such as solar panels, wind turbines, and battery storage systems.
These resources can be located anywhere on the network and can be connected to the network through various communication technologies.
Smart grids are driving the need for digital transformation, and data analytics is a critical tool for grid modernization efforts. By leveraging data analytics, utilities can build resiliency into the energy ecosystem and address vulnerabilities, while optimizing energy use and reducing costs.
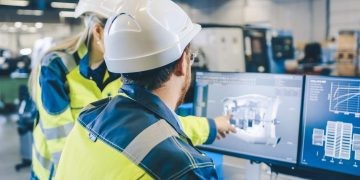
What role does advanced analytics play in identifying and mitigating risks in the energy transition?
The energy transition is a complex process that involves the adoption of new technologies and the integration of renewable energy sources into the existing electricity grid. This process carries a certain degree of uncertainty and risk, making risk identification and mitigation a critical part of the energy transition.
By applying advanced analytics in the energy transition, various risks can be identified and mitigated, such as:
Operational risks: The adoption of new technologies and the integration of renewable energy sources into the existing electrical grid can increase the complexity of the system and the probability of failures. Advanced analytics can be used to monitor and analyze operating data in real time to detect unusual patterns and prevent failures and errors.
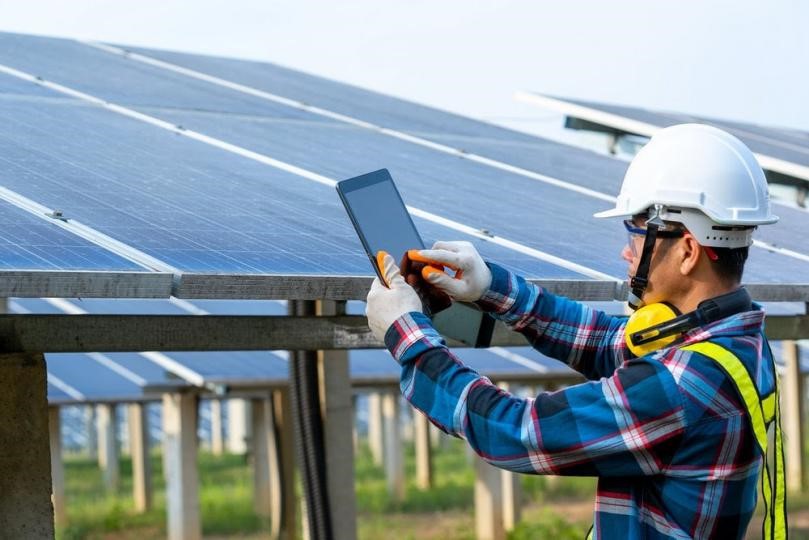
Regulatory risks: This energy transformation implies changes in the regulatory and political frameworks, which can generate uncertainty and risks for investors and system operators. Advanced analytics can help monitor and analyze regulatory and political changes to identify potential risks and opportunities.
Financial risks: This energy metamorphosis entails a high initial cost and a certain degree of uncertainty regarding the profitability of the investments. The application of evolved analytics can be used to assess the financial viability of projects and reduce uncertainty regarding the profitability of investments.
Climate risks: The energy transition aims to reduce greenhouse gas emissions and mitigate climate change. However, climate change can also create new risks for the electricity grid, such as increased temperatures and the intensity of storms.
How can engineers use advanced analytics to create customized and adaptive solutions for the Energy Transition?
Here are some of the top ways power engineers are able to use advanced analytics to create custom and adaptive solutions:
Modeling of energy systems: Energy experts can use advanced analytics to model and simulate energy systems. This allows them to identify problem areas and create custom solutions to address the specific challenges of each system.
T&D (Transmission and Distribution) systems modeling refers to the process of building mathematical models that represent the components and behavior of electrical power transmission and distribution systems.
These models can be used to simulate the behavior of electrical systems and predict their response to different operating conditions, such as variations in energy demand, system component failures, fluctuations in energy generation, among others.
T&D models can be extremely complex, as they include multiple components such as transformers, transmission lines, substations, switches, protections, etc. The models can also incorporate geographic, topographic, and meteorological data, making it possible to simulate the environmental conditions in which electrical systems operate.
Renewable energy optimization: This processing of complex events, it can be used to optimize the generation and storage of renewable energy. Energy engineers can use machine learning and artificial intelligence techniques to predict renewable energy production and optimize the operation of energy storage facilities.
Demand management: Some of these multivariate statistics can be used to manage consumer demand for energy. Energy engineers can use data analysis techniques to analyze energy consumption patterns and develop customized solutions to reduce energy consumption during times of high demand.
Monitoring and predictive maintenance: Energy experts can use advanced analytics to monitor the performance of energy systems and predict when maintenance or repairs are needed.
This allows power engineers to intervene before system failures occur and reduce maintenance costs.
Risk management: That sophisticated data analysis can be used to manage the risks associated with the energy transition. Power engineering specialists can use data analysis techniques to identify potential risks and develop custom solutions to mitigate them.
What opportunities are emerging for innovation in the field of energy as more and more data is collected and analyzed in real time?
As automation grows, data scientists will focus more on business needs, strategic monitoring, and deep learning. They will need to focus more on machine learning process operations, also called MLOps.
MLOps is a term that refers to the practices and tools used to manage and automate the lifecycle of machine learning models in production, from development and training to deployment, monitoring, and ongoing maintenance.
MLOps focuses on the automation and version control of models, as well as the management and monitoring of their performance in production. He is also concerned with the integration of models into business systems and applications and the collaboration between development, data science and operations teams.
Conclusion
The future of data analytics is poised for significant growth, fueled by technological advances, the rise of Big Data, and the growing importance of AI. New tools and techniques such as machine learning, natural language processing, and edge computing are constantly emerging, making data analysis more efficient and effective. Data scientists are needed in virtually every industry, not just technology.
References
Own source