Table of Contents
- Why is AI gaining relevance in NDT?
- The NDT technician and adaptation to AI
- Benefits of the use of artificial intelligence in advanced NDT techniques
- Robustness and generalization considerations of AI models.
- Explainability and interpretability of artificial intelligence models (XAI)
- Can AI replace the human inspector in NDT?
- Industrial AI applications in NDT
- AI performance evaluation metrics in NDT
- What is the main challenge in implementing AI in NDT?
- Conclusions
- References
Introduction
Non-destructive testing (NDT) is critical in industry in order to ensure the integrity and safety of components without compromising their functionality. Traditionally dependent on human expertise for data analysis and defect detection, the integration of artificial intelligence (AI) into NDT is driving unprecedented automation in industrial inspection.
AI increases accuracy, speeds up processes, optimizes costs and minimizes human error, a significant factor in signal and data interpretation. This article explores the influence of AI on nondestructive inspections, its applications, benefits, challenges and the future of inspection automation.
Why is AI gaining relevance in NDT?
Artificial intelligence is a considerable tool in non-destructive evaluation. Through this advanced technology, it is possible to optimize signal processing, accurately identify incipient structural damage and significantly improve decision making by applying machine learning algorithms that operate in real time on large volumes of dynamic data.
Additionally, the implementation of NDT with artificial intelligence in AI-enabled automated inspections (drones, robots, cameras) facilitates assessments in hard-to-reach areas, increasing coverage without sacrificing quality1. Autonomous, AI-enabled systems perform continuous inspections with minimal human intervention. In addition, AI enables automated real-time data analysis, which facilitates predictive maintenance scheduling, reduces downtime and improves operational efficiency.
The NDT technician and adaptation to AI
The non-destructive testing technician must be trained in both traditional inspection methodologies and the fundamentals of artificial intelligence. He/she must have solid knowledge in data interpretation, operation of classification algorithms and management of digital monitoring platforms. The NDT technician and adaptation to AI
This adaptation process requires constant updating of their skills, encompassing both the interpretation of analysis provided by AI and the operation of automated platforms to optimize industrial inspections. The modern NDT technician merges AI capabilities with their expertise, collaborating effectively with autonomous systems to improve the accuracy and efficiency of inspections.
Benefits of the use of artificial intelligence in advanced NDT techniques
AI optimizes the analysis of complex data in various non-destructive inspection techniques, driving advances in defect recognition and automatic classification:
- Ultrasound (PAUT/FMC): Automated analysis of complex signal data in advanced ultrasonic testing optimizes the detection and sizing of defects in heterogeneous materials and irregular geometries. In PAUT and FMC, convolutional neural networks (CNNs) such as U-Net segment defects in B-scan images, accurately quantifying their size and shape. Deep learning algorithms characterize defects by size, shape and orientation, and dynamically adapt scan parameters for superior coverage and sensitivity.
- Radiography (RT/CR/DR): AI optimizes the analysis of radiographic images to improve the detection of internal discontinuities such as cracks and porosities, with higher reliability and lower false positive rate. Architectures such as Faster R-CNN locate defects with high accuracy, and advanced AI processing reduces noise and improves the signal-to-noise ratio for better results.
- Thermography (TT): Automates the detection and analysis of thermal anomalies, identifying surface or subsurface defects and incipient equipment failures. Algorithms perform region of interest (ROI) segmentation and classify anomalies (electrical vs. mechanical problems), compensating for environmental variations for accurate analysis.
- Penetrating Liquids (PT): AI increases sensitivity in detecting surface discontinuities, even those of micrometer size using machine vision systems. Image processing techniques such as adaptive contrast enhancement and spatial filtering, combined with AI models, identify subtle patterns indicative of defects.
- Eddy Current (ECT/ECA): Improved detection of surface and subsurface defects in conductive materials with multi-frequency signal analysis. Recurrent neural networks (RNNs) and transformer architectures analyze sequences of ECT data, modeling complex signal responses to various defects and geometries. Modern algorithms interpret information locally, creating and combining thousands of pieces of data, where each can focus on a different image feature, improving defect detection and characterization.
In the following video, Acuren, a company dedicated to industrial inspection, explains how it is embracing digital transformation and artificial intelligence to optimize non-destructive testing. Its focus on safety is complemented by automation and data analytics to improve efficiency and reduce inspection costs, seeking a smarter and safer industry.
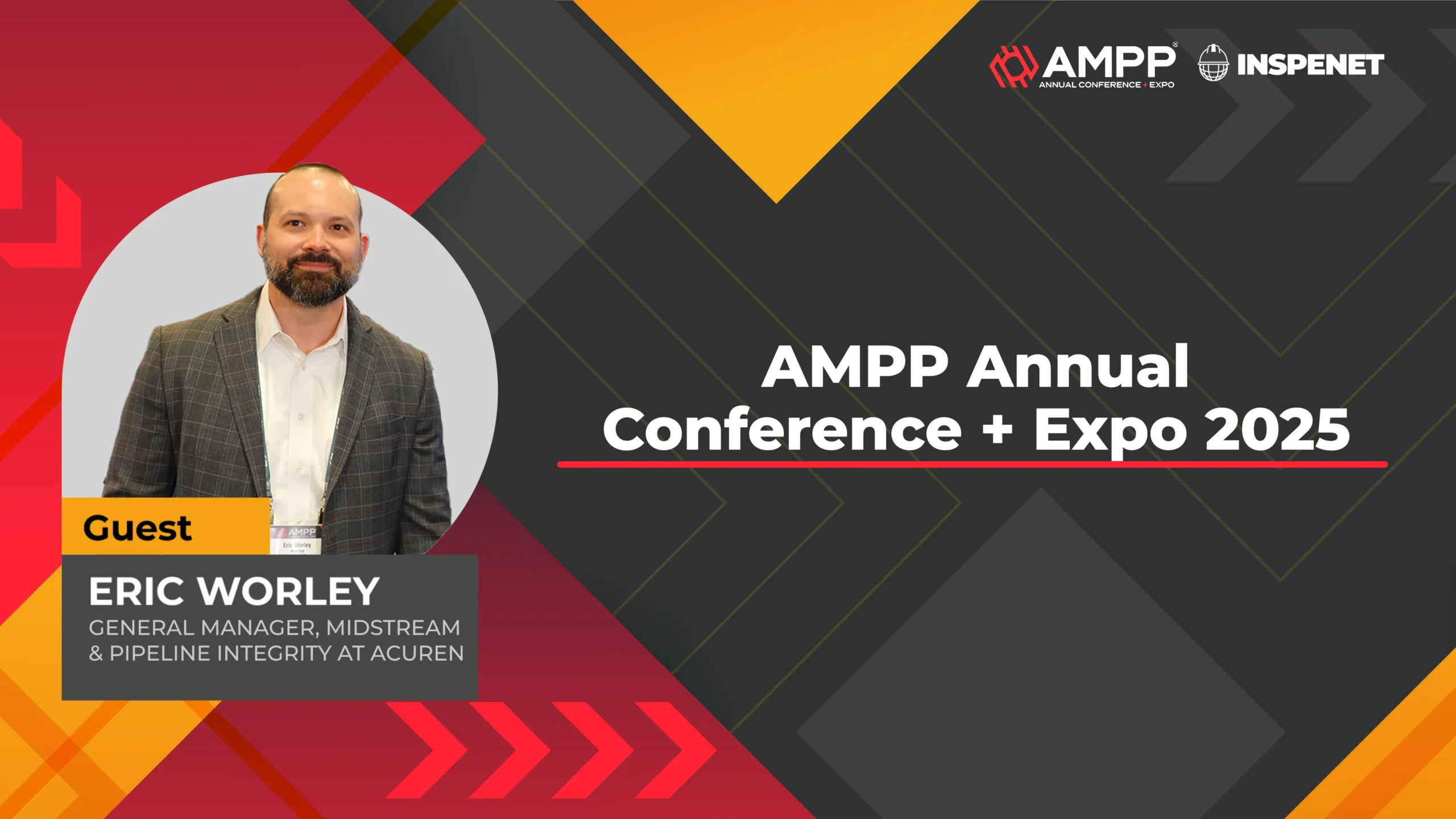
Robustness and generalization considerations of AI models.
The robustness of AI models in NDT is necessary for their performance in real industrial environments, where they must adapt to variations in input data, such as different industrial inspection equipment, operational variables or changing environmental conditions. Generalization, i.e., the model’s ability to identify new defects and analyze materials not seen during training, is another important challenge.
Techniques such as data augmentation, which generates synthetic variations of training data, and transfer learning, which reuses knowledge from similar tasks, are key strategies for improving robustness and generalization in automated data analysis for nondestructive testing.
Explainability and interpretability of artificial intelligence models (XAI)
The interpretability of AI models is critical in nondestructive testing applications, where decisions must be clear and fully understandable. Explainable Artificial Intelligence (XAI) seeks to increase transparency in decision-making processes by enabling the NDT technician to understand which parts of the input data influence the model prediction, which strengthens confidence in the results obtained and facilitates validation of the inspection process.
The integration of AI with advanced sensors and Internet of Things (IoT) platforms facilitates the creation of automated, real-time industrial inspection systems, including the use of robotic scanning. These systems enable continuous remote monitoring of critical infrastructure, such as bridges, gas pipelines, and industrial plants, where data collected by multimodal sensors is processed and analyzed using AI algorithms.
Through this analysis, early detection of anomalies is achieved, optimizing the identification of potential structural, corrosion or performance failures. AI-enabled systems generate warning signals before failures occur, thus improving operational reliability and minimizing unscheduled downtime.
Can AI replace the human inspector in NDT?
The concern about whether artificial intelligence could replace human inspectors in nondestructive testing is understandable. However, the reality is quite the opposite. AI does not replace the inspector, it represents a complementary work tool, automating repetitive tasks and optimizing data analysis. Despite improvements in data processing, the inspector’s experience and judgment are still needed to make final decisions, especially in complex situations.
The integration of advanced NDT techniques, artificial intelligence and robotization does not reduce the importance of the technician’s work; on the contrary, it emphasizes the need for highly trained professionals who can operate and understand this new equipment. As technology advances, so does the demand for technicians who can operate the tools, and who understand their limitations and potential; AI systems applied in NDT are designed to support and facilitate the inspector’s work, not to replace it.
Effective collaboration between humans and AI is key. Inspectors can use the analytics provided by AI to inform their decisions with greater accuracy and efficiency, ongoing training in these tools ensures that AI systems become strategic allies, reinforcing the inspector’s ultimate accountability and improving the overall quality of inspections.
Industrial AI applications in NDT
The integration of AI in non-destructive evaluation has enabled new industrial applications that improve the efficiency and safety of inspections:
- Autonomous inspection with drones and robots: AI-equipped drones and robots enable detailed inspections in hard-to-reach areas such as offshore platforms or high-rise structures. This improves accuracy and reduces risks for inspectors2.
- AI-based predictive maintenance: Algorithms can identify failure patterns in assets, anticipating problems and facilitating the programming of predictive models for condition-based maintenance.
- Maintenance digitization and transformation: The digitization of data generated by non-destructive inspections, combined with AI, enables the development of intelligent maintenance platforms that manage large volumes of data, optimize the traceability of activities and facilitate the integration of various data sources into a centralized and accessible system.
AI performance evaluation metrics in NDT
The efficacy of AI models in NDT is assessed by key metrics such as Accuracy, Recall, F1 Score, Area under the ROC curve (AUC) and the confusion matrix.
Although Probability of Detection (POD) is a metric used in non-destructive testing, its application is also influenced by advances in AI, as models can improve POD by identifying defects more accurately and reliably, thus optimizing compliance with inspection quality standards. The technological advances and digitization of the industrial revolution improve POD, with the ability of AI models to perform automated data analysis with greater accuracy.
What is the main challenge in implementing AI in NDT?
One of the main problems in implementing AI in non-destructive evaluation is the availability of large volumes of high-quality data to train the algorithms used. Collecting this data, especially in industries with obsolete equipment or in complex environments, can be an adversity.
The integration of AI with existing industrial systems requires investments and adaptation time, since modifying the equipment and processes in use implies operating costs; likewise, it is necessary to guarantee the reliability and traceability of the results obtained, ensuring that professionals are trained in the use of AI and promoting an organizational culture that values collaboration between AI and humans to optimize results.
Conclusions
Artificial intelligence is a transformational vector in nondestructive testing. Its integration, from automated inspection with AI to automated predictive data analysis and condition-based maintenance, redefines industrial asset integrity management. Despite the complexities related to its implementation, the inherent advantages are substantial.
AI-powered human-machine correlation optimizes accuracy and efficiency while opening new paradigms for industrial inspection. Continued research and development in interpretability, robustness and integration with advanced sensors projects a future of disruptive optimization for the NDT domain.
References
- Sharma, K., Kumar, A., & Sharma, K. (2023). Integration of robotics and artificial intelligence in non-destructive testing: A review of applications and challenges. International Journal of Creative Research Thoughts (IJCRT), 11(11), 285–295. https://www.researchgate.net/publication/372817358_Integration_of_Robotics_and_Artificial_Intelligence_in_Non-Destructive_Testing_A_Review_of_Applications_and_Challenges
- Mitchell, D., Blanche, J., Harper, S., Lim, T., Gupta, R., Zaki, O., Tang, W., Robu, V., Watson, S., & Flynn, D. (2022). A review: Challenges and opportunities for artificial intelligence and robotics in the offshore wind sector. Energy and AI, 8, 100146. https://doi.org/10.1016/j.egyai.2022.100146