Introduction
Industrial maintenance has evolved significantly in recent decades, moving from reactive, cyclical repair and replacement approaches to a more proactive model, based on advances in Reliability-Centered Maintenance (RCM) and data collection and analysis. This new approach has been driven by the accelerated advancement of digital technologies and Industry 4.0 and together they seek to optimize maintenance processes to improve safety, reduce costs, and increase operational efficiency.
What is intelligent industrial maintenance?
Intelligent Maintenance is defined as “a system that uses data analytics and decision support tools to predict and prevent potential machine failures1. Recent advances in information technology, computers, and electronics have facilitated the design and implementation of such systems. The key research elements of intelligent maintenance systems consist of:
- Transformation of data to information to knowledge and synchronization of decisions with remote systems.
- Intelligent and integrated forecasting algorithms to assess degradation and predict future performance.
- Software and hardware platforms to run online models.
- Integrated product services and lifecycle information for closed-loop product designs.
The concept is broader, so this article will cover both approaches.
We define intelligent industrial maintenance as maintenance that takes full advantage of the understanding of the logic of RCM and the technological advances that complement it, up to the benefits of the use of artificial intelligence.
It is convenient to begin by reviewing how RCM shows the new logic for viewing maintenance. Other articles have discussed the importance of understanding the six RCM Failure Patterns in order to design appropriate policies for managing asset failure modes. In summary, these failure patterns, mentioning that they conclude in three aspects of utmost importance:
1. That about 70% of the conditional probability of equipment failure is related to “Infant Mortality”. And the ways in which this high probability of failure can be prevented from becoming a failure is through two very clear policies. One is by taking care of all aspects related to the design, materials, and installation or assembly of the components.
It is important to use original parts (or substitutes of equal quality) and to monitor compliance with strict procedures for handling and assembly of parts (sequence, adjustments, torque, clearances, remaining misalignment, among others) with properly trained personnel and with adequate tools and work environment. The second way to avoid infant mortality is to avoid over-maintenance. That is, avoid performing maintenance at unnecessary frequencies (related to point 3) and try to be as minimally invasive as possible during maintenance.
2. About 11% of the failures respond to a conditional probability of failure related to “Wear and Tear” or known service life. This means that around this percentage, cyclic service or replacement tasks should be considered.
3. The 89% of failures respond to a conditional probability of random failures, so they do not benefit much from cyclic service or replacement tasks, and this is where the greatest benefit is obtained from predictive maintenance or condition-based maintenance.
A strategy that uses advanced technologies to continuously monitor the condition of industrial equipment and predict when maintenance intervention is needed. Unlike the cyclic maintenance described in the previous point, condition-based maintenance is triggered only when data indicates that a failure is imminent.
When analyzing the RCM Decision Tree we can observe that it privileges condition-based maintenance and this is totally in line with what was expressed in point 3 above. But it is also clear in this tree that it must be worthwhile to perform this type of maintenance, which could be costly. Of course, it also places the constraint of whether it is “worthwhile” to perform any other type of maintenance: cyclic repair or replacement, combination of tasks or fault-finding (for hidden failure modes). If none of these are worthwhile, even if technically feasible, the appropriate policy will be “Operate to Failure (RTF)”, although it clarifies that “A change may be desirable”.
Key components of smart industrial maintenance
We consider intelligent maintenance to be anything that supports us in reducing downtime, optimizing costs, increasing equipment reliability, improving safety, increasing equipment life and improving energy efficiency. To achieve these objectives, intelligent maintenance must have the following components:
- Avoid being affected by infant mortality: Take care to perform maintenance in the best possible way, avoiding that we have to put them into operation with a rosary in our hands.
- Avoid over-maintenance, both in frequency and invasive intensity, on the equipment. In other words, “leave still what is still”.
- Privilege maintenance by condition or “Predictive Industrial Maintenance” over any other policy, although its cost-risk-benefit justification will always be required. Within this maintenance we will find different condition monitoring devices, with which we will perform “trend analysis”.
- Selecting the right condition devices: A thorough study of the intervals and P-F curves will give us valuable information on how to select the right condition devices. For example, conveyor belt idlers or low-criticality electric motors will probably justify a thermal imaging camera to capture the temperature increase of their bearings, a phenomenon that is in the middle of the P-F curve.
Whereas bearings in critical equipment will likely warrant monitoring with ultrasound, lubricant analysis or vibration to detect defects more towards the beginning of the P-F curve.
- Monitoring devices: In terms of condition monitoring, the appropriate types must be located according to the asset under analysis. Tied to this we will find multiple tools on the market where, fortunately, these have come down considerably in price and have become more efficient. Such as the case of IoT Sensors, which collect real-time data on the condition of equipment, such as vibrations, temperature, pressure, and power consumption. An excellent guide for selecting condition monitoring techniques and tools is provided in John Moubray’s RCMII book2 in Annex 4.
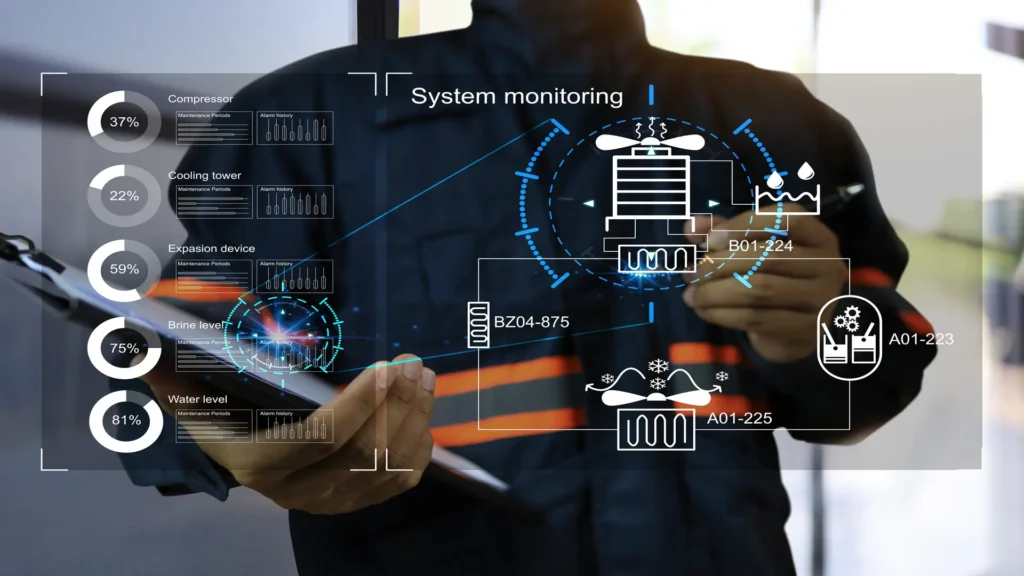
- Operate the failure: It will be the appropriate policy for those failure modes where no preventive effort is worthwhile (including cyclic repair or replacement, inspection, condition monitoring or fault-finding).
- Rely on artificial intelligence: One way to rely on AI is that it processes and analyzes data collected by sensors, using artificial intelligence and machine learning algorithms to identify patterns and detect anomalies and their proper diagnosis.
- Management platforms: Such as CMMS (SAP-PM or Maximo type) or APMs (Meridium type) that centralize information and allow technicians and managers to visualize the status of equipment, generate alerts, plan maintenance interventions, keep indicators and rely on failure analysis tools, among others.
- Data as a central element of maintenance: Data that is first collected from the equipment through IoT and then analyzed to enable predictive maintenance to react quickly and make the right decisions. This aims to optimize technician interventions and reduce equipment downtime.
Conclusions
Intelligent industrial maintenance represents the complementation between the selection of appropriate policies to avoid failures, data platforms, management, indicators, and adequate analysis coupled with a revolution that is underway and will not stop in the way companies manage their assets.
Organizations and companies through the use of digital technologies, artificial intelligence, and machine learning can increase efficiency, reduce costs, and improve the reliability of their operations. As the industry continues to evolve, intelligent maintenance will become an essential component to ensure competitiveness in the global marketplace.
References
- https://en.wikipedia.org/wiki/Predictive_maintenance
- John Moubray; “RCM2 Reliability Centered Maintenance.”