Table of Contents
- Introduction
- The technological leap of RBI: From matrices to dynamic models
- Emerging technologies in tank RBI: Tools leading the change
- Technical validation under API 653 for the normative applicability of PAUT and PEC in tanks
- Robotics applied to RBI: Inspection without interruptions
- Integration of digital platforms for RBI
- Simulated technical case: Digitized RBI in an API 650 tank
- The Future of RBI in the storage industry: Toward a predictive culture
- Conclusions
- References
Introduction
The article published by INSPENET, titled “Reliability in Storage Tanks through RBI“, clearly establishes the regulatory and operational foundations that support Risk Based Inspection (RBI) in atmospheric tanks, emphasizing the reference framework of API RP 580 and API 653 standards. This approach has proven to be key in optimizing resources, reducing unnecessary shutdowns, and improving the operational reliability of storage assets.
However, today’s operational context—marked by demanding production cycles, highly corrosive environments, and increasingly stringent regulatory requirements—demands going beyond mere compliance. Nowadays, the most effective RBI programs are not limited to static risk matrices but integrate emerging technologies, digital platforms, predictive modeling, and specialized robotics to develop intelligent inspection and maintenance strategies.
This new approach aims to expand the scope of the original publication by offering professionals in the field concrete tools, advanced risk assessment methods, optimized maintenance plans, and representative cases that demonstrate how RBI can evolve into a dynamic platform for managing the mechanical integrity of storage tanks.
The technological leap of RBI: From matrices to dynamic models
Since its widespread adoption, Risk Based Inspection (RBI) has largely relied on the construction of matrices that combine two key variables: Probability of Failure (PoF) and Consequence of Failure (CoF). While these matrices are useful, they tend to be static, temporally insensitive, and often based on qualitative or semi-quantitative criteria that do not accurately reflect the dynamic conditions of operation.
The evolution of the RBI approach is well illustrated in the following video, which serves as an ideal learning capsule to contextualize the shift toward dynamic models (courtesy of Equity Technology Group).

What is Risk-Based Inspection?
The major technological leap in the evolution of RBI lies in the integration of real-time operational datfa, multivariable historical datasets, and advanced deterioration models that enable the continuous updating of a tank’s risk profile. This evolution has led to what is now known as intelligent RBI—an approach that incorporates data analytics, digital platforms, digital tools, digital twins, and systems that simulate the future behavior of assets under various operational or environmental scenarios.
This paradigm shift, framed within the context of industrial digital transformation, enables a proactive and predictive management approach where risk is not just a static estimate but a variable that evolves with every new data point, continuously reinforcing the risk assessment of the inspected assets. Transitioning to dynamic models not only improves the accuracy of integrity assessments but also enhances the efficiency of maintenance plans aligned with real-time asset conditions.
Emerging technologies in tank RBI: Tools leading the change
The transformation of Risk Based Inspection (RBI) in atmospheric storage tanks cannot be envisioned without the integration of advanced technologies that enhance data accuracy, reduce uncertainty, and feed more representative deterioration models. Below are the key emerging tools that are redefining integrity management in tanks:
- 3D Laser Scanning: This technique enables high-precision three-dimensional mapping of structures such as tank bottoms, annular plates, and shells. Laser scanning detects settlement, buckling, or deformations that may compromise the tank’s geometric stability. When integrated with geometric modeling software, these scans become a foundation for predictive structural analysis or deviation comparisons using digital twin baselines.
- Phased Array Ultrasonic Testing (PAUT): PAUT enables angular and sectorial sweeps across critical areas of the tank bottom and shell, offering high-resolution detection of localized corrosion, cracking, and wall loss. Unlike conventional UT, Phased array ultrasonic testing (PAUT) provides broader coverage per pass, higher sensitivity, B/C-scan imaging, and better detection in complex geometries.
- Pulsed Eddy Current (PEC): This electromagnetic technique does not require direct contact with the base metal. It is ideal for evaluating tank bottoms under coatings or in inaccessible areas without the need to remove linings. It enables wall thickness measurement and hidden defect detection, reducing surface preparation costs.
- Digital Twin + RBI: Digital twins allow simulation of deterioration scenarios, prediction of mechanical behavior under changing loads and environments, and real-time adjustment of RBI plans. When virtual simulation is linked with real inspection and operational data via digital platforms, the result is a dynamic structural integrity platform that facilitates data-driven decisions, real-time risk assessment, and complete traceability.
Technical validation under API 653 for the normative applicability of PAUT and PEC in tanks
The implementation of advanced technologies in Risk Based Inspection (RBI) programs requires a clear understanding of the regulatory framework that governs them—or, in some cases, allows them by omission. In the specific case of atmospheric storage tanks governed by the API 653 standard, a common field question arises: Is it acceptable to use techniques such as PAUT (Phased Array Ultrasonic Testing) or PEC (Pulsed Eddy Current) without violating the standard?
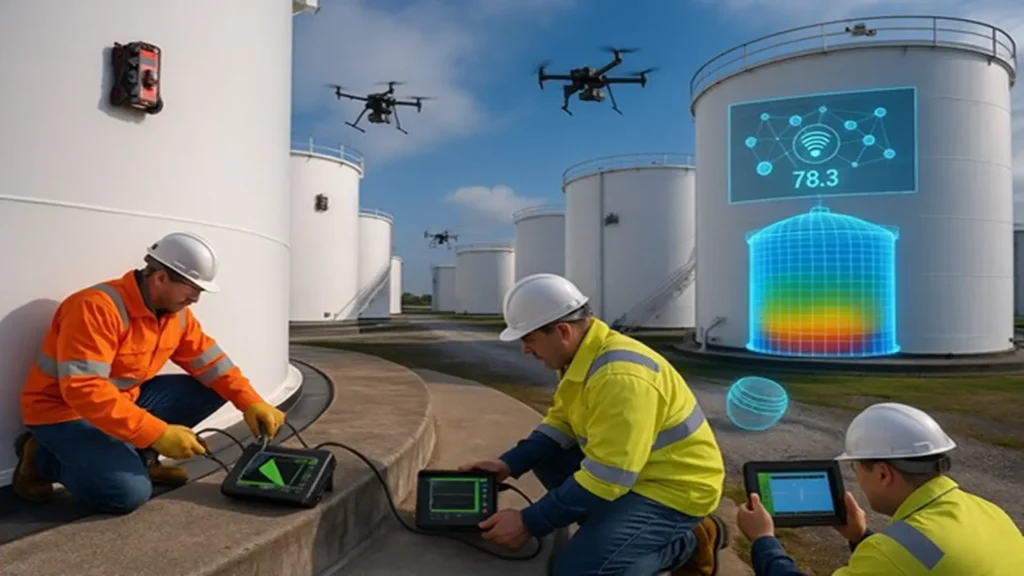
Answering this requires a careful reading of the standard. API 653 mandates the use of ultrasonic thickness measurement techniques, especially for inspections of the bottom, shell, and other critical areas. However, it does not specify that these measurements must be performed exclusively using conventional straight-beam UT. This regulatory openness allows, under engineering judgment, the use of advanced methods like PAUT, provided that:
- The inspection objective (thickness measurement, metal loss detection, discontinuity identification) is met with equal or greater precision.
- The procedure is qualified under recognized standards (SNT-TC-1A, ISO 9712, API 577).
- Personnel are properly certified.
- The results are traceable in RBI records and documented with B-scan or S-scan images.
PEC, on the other hand, is explicitly mentioned in the latest editions of API 653 as an appropriate technique for inspecting coated bottoms or surfaces where contact preparation is unfeasible. Its ability to detect wall loss through coatings makes it ideal for non-intrusive inspection and deterioration monitoring in critical areas such as annular plates.
In the context of RBI, both PAUT and PEC are not only technically valid but also enhance the reliability of PoF models through more accurate characterization of actual deterioration. Their use is fully justified when transitioning from a compliance-based inspection to a smart, predictive approach without compromising the guidelines of API 653 or API RP 580.
Robotics applied to RBI: Inspection without interruptions
One of the most disruptive innovations in RBI programs for atmospheric tanks is the use of autonomous robotic systems capable of inspecting the interior of tanks without the need to take them out of service. This solution eliminates high risk tasks for personnel, drastically reduces downtime, and ensures robust data capture to feed risk models.
Companies like Square Robot, Eddyfi Technologies, and Deep Trekker have developed first- and second-generation robots capable of autonomously navigating inside full tanks. These units are equipped with multiple technologies such as:
- Ultrasonic Testing (UT) and PAUT for thickness measurement and defect detection
- PEC for under-coating evaluations
- High definition cameras for remote visual inspection
- Navigation sensors and real-time mapping algorithms.
The data collected by these robots not only meets regulatory requirements but also feeds dynamic RBI models, updating PoF/CoF levels and continuously strengthening risk assessment based on recent and reliable information.
Among documented real-world cases, robotic inspection has been successfully implemented in crude oil storage terminals in the United States and the Middle East. These applications have extended internal inspection intervals by up to 40%, reduced cleaning and ventilation costs by more than 60%, and eliminated the need for personnel entry into confined spaces.
Robotics is no longer a futuristic option, it is a present and functional tool in modern RBI programs, supporting safer and more cost effective maintenance plans.
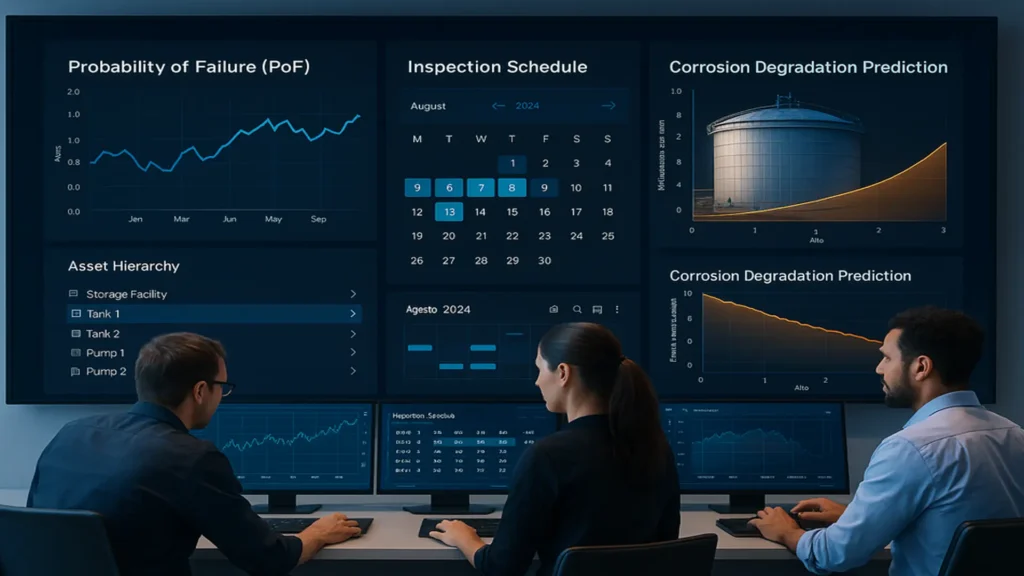
Integration of digital platforms for RBI
The digitalization of RBI processes is the central pillar for scaling these strategies in high-volume operations. Specialized platforms such as Meridium (GE Digital), Axxim (DNV), or PCMS (Antea) enable the consolidation of historical asset data, inspection results, risk analysis, and standardized technical documentation (API, ASME, AMPP) into a single interface.
These systems allow users to:
- Configure individualized risk profiles for each asset.
- Establish automated inspection plans based on quantitative PoF/CoF analysis.
- Integrate field sensor data (IoT) and advanced NDT tools.
- Generate visual dashboards that display, in real time, the integrity status of the facility, the evolution of risk, and required actions.
In addition, these platforms easily integrate with maintenance systems (CMMS), facilitating predictive decision-making and reducing unnecessary interventions. In this context, strengthening technical capabilities, data interoperability, and digital culture is essential for integrity teams to fully leverage the potential of these tools.
Simulated technical case: Digitized RBI in an API 650 tank
Imagine a storage terminal located in a coastal industrial area operating a flat-bottom API 650 tank with a capacity of 50,000 barrels, used for gasoline storage. The equipment, in service for over 12 years, has a mixed history of conventional inspections and minor repairs in the annular region.
To extend the internal inspection interval and optimize resources, a digitized RBI program is implemented as part of the asset’s integrity master plan. The first step involves conducting a 3D laser scan of the tank bottom and annular plate, enabling detection of deformations caused by differential settlement. Next, PAUT is applied to critical areas of the bottom and the first course of the shell to assess wall loss, cracks, and localized corrosion with high resolution.
The collected data is integrated into digital RBI platforms (e.g., Meridium or PCMS), which combine the historical PoF/CoF profile with the newly acquired technical evidence. As a result, an optimized inspection interval table is generated, allowing the next internal inspection to be extended by an additional 5 years, cutting shutdown costs by more than 40%, and technically justifying the decision before regulatory bodies. Operational risk analysis is reduced by 60%, and operations personnel gain clear visibility into the tank’s structural condition.
The Future of RBI in the storage industry: Toward a predictive culture
The evolution of Risk Based Inspection (RBI) in the storage industry does not rely solely on the adoption of new technological tools, but rather on a deep cultural transformation. Traditional practices based on fixed inspection schedules or reactive decision-making have proven costly, inefficient, and, in many cases, insufficient for effective risk assessment capable of preventing catastrophic failures.
The future of RBI is closely linked to the consolidation of a predictive culture, where decisions are based on probabilistic deterioration analysis, dynamic consequence evaluation, and the continuous integration of data from advanced inspections, remote sensors, and digital modeling.
This shift requires maintenance, mechanical integrity, and operations professionals to develop new technical and analytical competencies, to understand the language of risk, and to interpret data that allows them to anticipate critical events. It also calls for a restructuring in how inspections are planned, prioritized, and executed—allocating resources strategically and justifiably.
Adopting a risk-based mindset is not just about complying with standards it means operating with technical intelligence. In this new stage, RBI is no longer just a diagnostic tool, but a proactive decision-making platform that guides organizations toward greater reliability, efficiency, and operational safety.
Conclusions
Risk Based Inspection (RBI) has evolved from a static model based on matrices into a dynamic decision-making system driven by data, multivariable analysis, and disruptive technologies. In the context of atmospheric tanks, this shift not only leads to better technical and economic outcomes but also reflects a more mature approach to structural integrity and risk management.
This analysis confirms that, while standards such as API RP 580 and API 653 remain essential pillars, the incorporation of tools like 3D scanning, RBI platforms, specialized robotics, PAUT, and PEC significantly expands the value of these frameworks. In particular, it validates that although PAUT is not explicitly mentioned in API 653, its use is technically valid under sound engineering criteria, while PEC is explicitly approved.
The next step for the industry is not just implementing RBI it is understanding it as a living, connected, and constantly evolving system that is fueled by real data, updated with each inspection, and enables maintenance plans to be adjusted based on actual risk, rather than static assumptions. This is achieved by integrating digital platforms, advanced technologies, and a technical culture rooted in predictive analysis.
References
Own source