Introduction
The oil and gas industry constantly faces significant operational risks that can result in substantial economic losses, catastrophic environmental damage and, most importantly, endanger human lives. From offshore rigs to FPSO (Floating Production Storage and Offloading) units to pipelines and refineries, each segment of the value chain presents unique risk management challenges.
In this highly complex context, artificial intelligence (AI) and machine learning are revolutionizing the way these risks are identified, assessed and mitigated. This article explores the fundamental purpose of operational risk assessment in the industry and how new AI-based technologies are transforming this critical field.
The primary objective of operational risk management
Operational risk management in the oil industry is primarily aimed at protecting the organization’s critical assets – people, environment, facilities and reputation – while ensuring operational continuity and optimizing financial performance.
Specific objectives
- Incident prevention: Identifying potential failures or hazardous conditions before they lead to accidents or incidents.
- Regulatory compliance: Ensure operations comply with increasingly stringent local and international regulations.
- Cost optimization: Reduce losses from unplanned outages, reactive maintenance or regulatory fines.
- Environmental protection: Prevent spills, uncontrolled emissions or other negative environmental impacts.
- Personnel safety: Ensure a safe working environment for all employees and contractors.
- Operational sustainability: Maintain social license to operate through responsible risk management practices.
In the specific context of upstream operations, such as offshore platforms and FPSO units, these targets take on an additional dimension of complexity due to the hostile environment, the remoteness of the facilities and the high concentration of potential risks and cyberattacks.
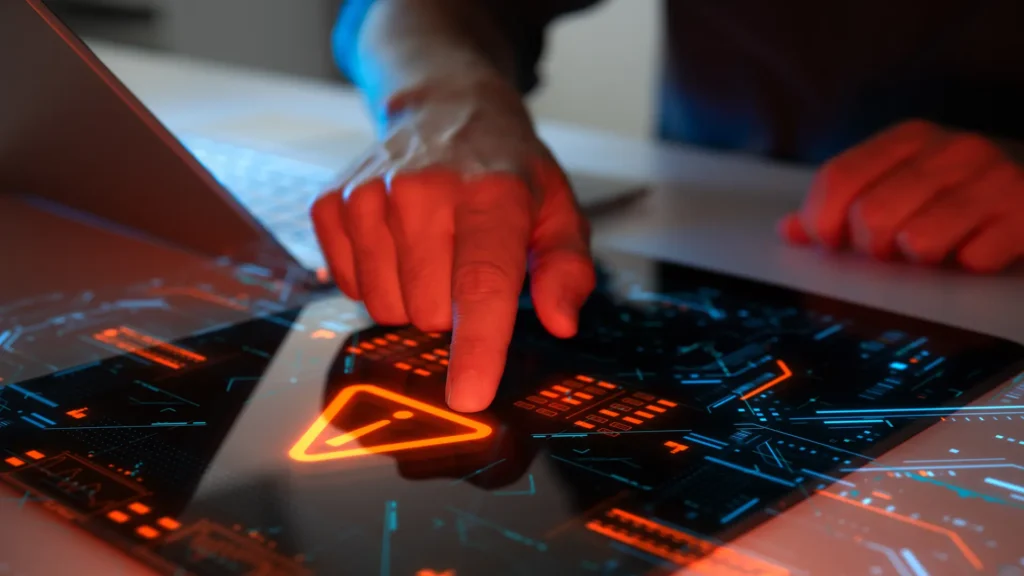
Contributions of AI to operational risk identification and analysis
Artificial intelligence (AI) has become a key element for operational risk management in the oil and gas industry, providing innovative solutions to anticipate, identify and mitigate risks at all stages of the value chain. The main contributions are summarized below:
Advanced predictive analytics
AI and machine learning have radically transformed predictive capabilities in the oil industry:
- Predictive maintenance: Algorithms that analyze real-time sensor data from critical equipment such as compressors, pumps and turbines can detect subtle anomalies that predict failures before they occur, especially important on offshore platforms where component replacement is logistically complex and costly.
- Predicting risk events: Deep learning models that, fed with historical data on incidents, operating conditions and environmental factors, can identify complex patterns that precede high-risk situations in FPSO units and other offshore installations.
Real-time monitoring
- Intelligent surveillance systems: AI video analysis to detect abnormal behavior, presence of personnel in restricted areas or risky conditions in extraction platforms.
- Smart sensors and IoT: Networks of interconnected sensors that monitor critical variables such as pressure, temperature and chemical composition, enabling immediate detection of dangerous deviations in pipelines and process equipment.
- Digital twins: Virtual representations of physical facilities such as FPSOs that allow simulating risk scenarios and evaluating mitigation measures before implementing them.
Emergency response optimization
- Emergency simulation: Algorithms that model and predict the development of emergencies such as fires, explosions or spills on offshore platforms, facilitating the planning of optimized responses.
- Dynamic evacuation routes: AI systems that recalculate evacuation routes in real time according to the evolution of an emergency in offshore installations.
Integrated value chain analysis
Systemic risk monitoring: Identification of interdependencies and cascading effects between different segments of the oil value chain, from extraction (upstream) to transportation (midstream) and refining (downstream).
Operation optimization: Algorithms that balance safety and operational efficiency, especially critical in maritime operations where margins of error are minimal.
Exploration and production optimization
- AI analyzes large volumes of seismic, geological and sensor data to identify potential reservoirs and optimize drilling strategies, reducing geological and operational risks.
- Companies such as ExxonMobil and Chevron have demonstrated improvements in reservoir prediction accuracy and drilling planning, reducing risk exposure and improving profitability.
Critical infrastructure monitoring and management
- AI platforms enable remote monitoring of pipelines and other infrastructure, detecting leaks, corrosion and structural weaknesses early.
- Proactive monitoring with AI helps minimize the environmental impact and risks associated with failures in hydrocarbon transportation systems.
Spill detection and prevention
- AI processes satellite data, radar images and underwater sensors to quickly identify oil spills, facilitating an immediate and effective response that reduces environmental and economic damage.
- Advanced predictive and simulation models can anticipate the spread of spills and design more effective containment strategies.
Regulatory compliance and risk assessment
- AI automates data collection and analysis to ensure compliance with environmental and safety regulations, assessing risks in real time and improving responsiveness to regulatory changes.
- This strengthens companies’ ability to operate within legal standards and minimize penalties.
Benefits summary
Contribution of AI | Impact on operational risk management |
---|---|
Early risk detection | Reducing accidents and improving safety |
Predictive maintenance | Fewer failures, less downtime |
Exploration and production optimization | Reduction of geological and operational risks |
Infrastructure monitoring | Leak and structural failure prevention |
Spill detection | Rapid response and mitigation of environmental damage |
Regulatory compliance | Reduced exposure to sanctions and improved reputation |
Logistics optimization | Supply chain risk reduction |
In this context, the use of innovative technology in operational risk management, generative AI, can revolutionize the way organizations process and use information, offering advanced analytics, accurate predictions and automated solutions.
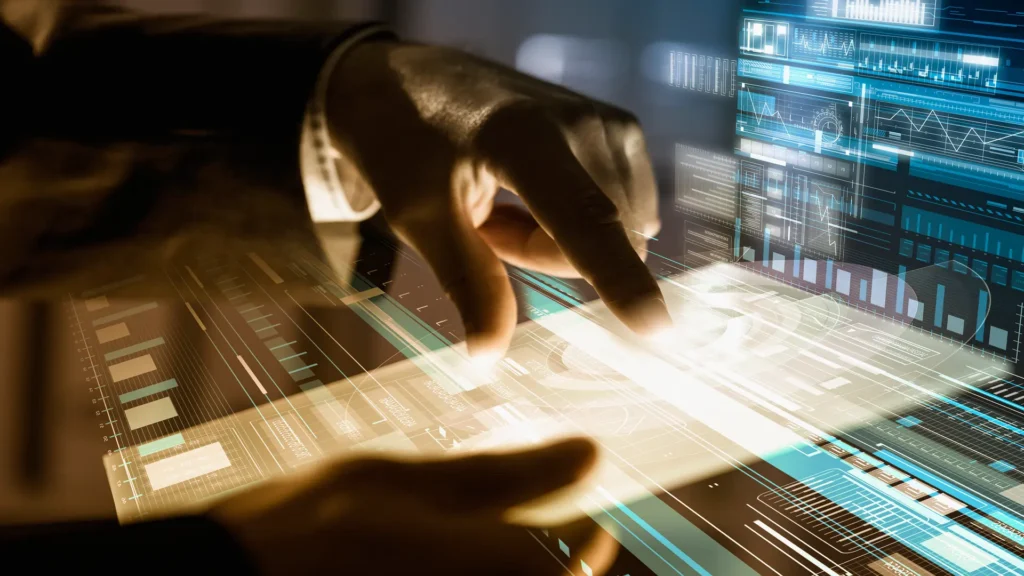
Specific applications by industry segment
Upstream (Exploration and production)
On offshore platforms and FPSO units, AI is revolutionizing risk management through:
- Advanced seismic analysis: Deep learning algorithms that improve the interpretation of seismic data, reducing geological risks during drilling.
- Structural integrity monitoring: Sensors combined with AI that detect material fatigue, corrosion or structural damage on offshore platforms before they pose a danger.
- Drilling optimization: Systems that analyze real-time data during drilling operations to prevent blowouts and other critical incidents.
- Control of dynamic positioning systems: Advanced algorithms that maintain the safe position of FPSO units in adverse sea conditions.
Midstream (Transportation and storage)
- Pipeline leak detection: Anomaly algorithms that detect micro-leaks before they become major problems by analyzing pressure and flow data.
- Shipping route optimization: Systems that consider climatic, geopolitical and logistical factors to minimize risks during the transport of crude oil by sea.
- Tank integrity monitoring: Solutions that combine drone inspections and AI image analysis to detect structural problems in storage tanks.
Downstream (Refining and distribution)
- Advanced process control: Algorithms that optimize refining operations in real time, keeping critical variables within safe ranges.
- Emissions management: AI-based monitoring systems that prevent hazardous emissions through early detection of deviations in refining processes.
- Risk scenario simulation: Models that evaluate the potential impact of different operational configurations on risks in refining facilities.vvvv
Challenges and considerations
- Data quality and availability: The performance of AI systems is directly dependent on the quality of the data used to train them. In environments such as offshore platforms, where conditions can be extreme, ensuring data integrity and consistency represents a significant challenge.
- Model interpretability: For safety-critical decisions, such as those made on FPSO units or during deepwater drilling operations, the ability to understand why an algorithm has reached a certain conclusion is critical.
- Integration with existing systems: Implementation of AI solutions must consider the existing technology infrastructure, particularly in older installations where a complete upgrade can be prohibitively expensive.
- Human factor: The interaction between human operators and AI systems requires careful consideration. Overreliance or, conversely, distrust of automated recommendations may introduce new operational risks.
Success stories
- Predictive system on North Sea platform: An international oil company implemented an AI-based predictive maintenance system that analyzes more than 40,000 data points per second from an offshore platform. The system identified early impending failures on a critical compressor that would have caused an unplanned shutdown at an estimated cost of $2 million per day. Early detection enabled scheduled maintenance with minimal operational disruption.
- Safety optimization at Brazilian FPSO: An FPSO operator in Brazil implemented an AI-based monitoring system that integrates meteorological, operational and structural data. During a severe weather event, the system recommended specific operational modifications that prevented potential structural damage, avoiding risks to personnel and potential spills.
- Risk reduction at North American refinery: A refinery implemented an AI system to optimize its critical processes, achieving a 35% reduction in recordable safety events and a 22% decrease in unscheduled emissions, as well as significant improvements in energy efficiency.
Future trends
- Autonomous systems: Progressive implementation of systems that not only detect risks but also take autonomous corrective actions, especially important in remote installations such as offshore platforms.
- Federated AI: Development of systems that enable collaborative learning between different facilities without sharing sensitive data, crucial for companies with global operations.
- Blockchain integration: Implementation of immutable records for safety-critical data, ensuring traceability of decisions and actions throughout the value chain.
- Augmented and virtual reality: Convergence of AI with visualization technologies to improve training and emergency response in high-risk environments such as FPSO units.
Conclusions
Digital transformation through artificial intelligence (AI) and machine learning is redefining operational risk management in the oil and gas industry. These technologies do not replace human expertise but rather enhance it by offering advanced analytical capabilities to process real-time data, detect abnormal behavior patterns, and predict potential failures. In highly hazardous UPSTREAM environments—such as offshore platforms and FPSO units—AI has proven invaluable for proactive incident prevention, significantly reducing exposure to critical events.
In MIDSTREAM and DOWNSTREAM operations, intelligent systems have enabled a holistic risk perspective across the entire value chain—from transportation and storage to refining and distribution. The integration of operational data from sensors, SCADA, ERP systems, and others enables a more comprehensive and dynamic approach to risk analysis. This not only improves response to contingencies but also raises standards for regulatory compliance, energy efficiency, and occupational safety in complex facilities.
Strategic adoption of AI within risk management frameworks translates into a sustainable competitive advantage for companies in the sector. Organizations that integrate these tools early will not only optimize the reliability and availability of critical assets but also be better equipped to address emerging market challenges—such as regulatory pressure, environmental demands, and increasing operational complexity. This shift is not optional but a necessity to ensure safe, resilient, and sustainability-aligned operations.
References
- https://inspenet.com/articulo/la-deteccion-de-derrames-de-petroleo-con-ia/
- https://www.ultralytics.com/es/blog/ai-in-oil-and-gas-refining-innovation
- https://www.mordorintelligence.com/es/industry-reports/ai-market-in-oil-and-gas
- https://www.chetu.com/es/blogs/oil-gas/ai-machine-learning-oil-gas-transformation.php
- https://es.t-mobile.com/business/resources/articles/oil-and-gas-digital-transformation